Revisiting The Relationship Between Internal and External Validity
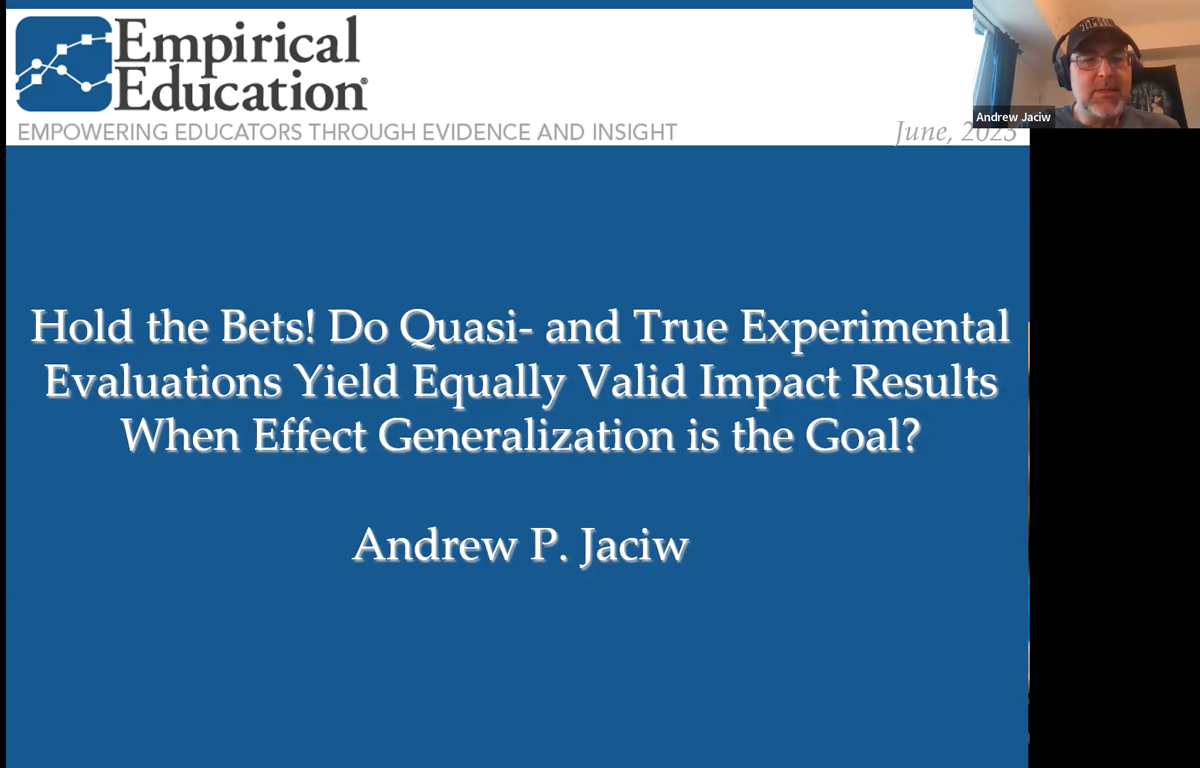
The relationship between internal and external validity has been debated over the last few decades.
At the core of the debate is the question of whether causal validity comes before generalizability. To oversimplify this a bit, it is a question of whether knowing “what works” is logically prior to the question of what works “for whom and under what conditions.”
Some may consider the issue settled. I don’t count myself among them.
I think it is extremely important to revisit this question in the contemporary context, in which discussions are centering on issues of diversity of people and places, and the situatedness of programs and their effects.
In this blog I provide a new perspective on the issue, one that I hope rekindles the debate, and leads to productive new directions for research. (It builds on presentations at APPAM and SREE.)
I have organized the content into three degrees of depth. 1. For those interested in a perusal, I have addressed the main issues through a friendly dialogue presented below. 2. For those who want a deeper dive, I provide a video of a PowerPoint in which I take you through the steps of the argument. 3. The associated paper, Hold the Bets! Do Quasi-and True Experimental Evaluations Yield Equally Valid Impact Results When Effect Generalization is the Goal?, is currently posted as a preprint on SAGE Advance, and is under review by a journal.
Lastly, I would really value your comments to any of these works, to keep the conversation, and the progress in and beneficence from research going. Enjoy (and I hope to hear from you!),
Andrew Jaciw
The Great Place In-Between for Researchers and Evaluators
The impact evaluator is at an interesting crossroads between research and evaluation. There is an accompanying tension, but one that provides fodder for new ideas.
The perception of doing research, especially generalizable scientific research, is that it contributes information about the order of things, and about the relations among parts of systems in nature and society, that leads to cumulative and lasting knowledge.
Program evaluation is not quite the same. It addresses immediate needs, seldom has the luxury of time, and is meant to provide direction for critical stakeholders. It is governed by Program Evaluation Standards, of which Accuracy (including internal and statistical conclusions validity) is just one of many standards, with equal concern for Propriety and Stakeholder Representation.
The activities of the researcher and the evaluator may be seen as complementary, and the results of each can serve evaluative and scientific purposes.
The “impact evaluator” finds herself in a good place where the interests of the researcher-evaluator and evaluator-researcher overlap. This zone is a place where productive paradoxes emerge.
Here is an example from this zone. It takes the form of a friendly dialogue between an Evaluator-Researcher (ER) and a Researcher-Evaluator (RE).
ER: Being quizzical about the problem of external validity, I have proposed a novel method for answering the question of “what works”, or, more correctly of “what may work” in my context. It assumes a program has not yet been tried at my site of interest (the inference sample), and it involves comparing the performance across one or more sites where the program has been used, to performance at my site. The goal is to infer the impact for my site.
RE: Hold-on. So that’s kind of like a comparison group design but in reverse. You’re starting with an untreated group and comparing it to a treated group to draw an inference about potential impact for the untreated group. Right?
ER: Yes.
RE: But that does not make sense. That’s not the usual starting point. In research we start with the treated group and look for a valid control, not the other way around. I am confused.
ER: I understand, but when I was teaching, such comparisons were natural. For example, we compared the performance of a school just like ours, but that used Success For All (SFA), to performance at our school, which did not use SFA, to infer how we might have performed had we used the program. That is, to generalize the potential effect of the program for our site.
RE: You mean to predict impact for your site.
ER: Call it what you will. I prefer generalize because I am using information about performance under assignment to treatment from somewhere else.
RE: Hmmm. Odd, but OK (for now). However, why would you do that? Why not use an experimental result from somewhere else, maybe with some adjustment for differences in student composition and other things? You know, using reweighting methods, to produce a reasonable inference about potential impact for your site.
ER: I could, but that information would be coming from somewhere else where there are a lot of unknown variables about how that site operates, and I am not sure the local decision-makers would buy it. Coming from elsewhere it would be considered less-relevant.
RE: But your comparison also uses information from somewhere else. You’re using performance outcomes from somewhere else (where the treatment was implemented) to infer how your local site would have performed had the treatment been used there.
ER: Yes, but I am also preserving the true outcome in the absence of treatment (the ‘business as usual’ control outcome) for my site. I have half the true solution for my site. You’re asking me to get all my information from somewhere else.
RE: Yes, but I know the experimental result is unbiased from selection into conditions at the other “comparison” site, because of the randomized and uncompromised design. I‘ll take that over your “flipped” comparison group design any day!
ER: But your result may be biased from selection into sites, reflecting imbalance on known and possibly unknown moderators of impact. You’re talking about an experiment over there, and I have half the true solution over here, where I need it.
RE: I’ll take internal validity over there, first, and then worry about external validity to your site. Remember, internal validity is the “sine qua non”. Without it, you don’t have anything. Your approach seems deficient on two counts: first from lack of internal validity (you’re not using an experiment), and second from a lack of external validity (you’re drawing a comparison with somewhere else).
ER: OK, now you’re getting to the meat of things. Here is my bold conjecture: yes, internal and external validity bias both may be at play, but sometimes they may cancel each other out.
RE: What!? Like a chancy fluky kind of thing?
ER: No, systematically, and in principle.
RE: I don’t believe it. Two wrongs (biases) don’t make a right.
ER: But the product of two negatives makes a positive.
RE: I need something concrete to show what you mean.
ER: OK, here is an instance… The left vertical bar is the average impact for my site (site N). The right vertical bar is the average impact for the remote site (site M). The short horizontal bars show the values of Y (the outcome) for each site. (The black ones show values we can observe, the white-filled one shows an unobserved value [i.e., I don’t observe performance at my site (N) when treatment is provided, so the bar is empty.]) Bias1 is the difference between the other site and my site in the average impact (the difference in length of the vertical bars). Bias2 results from a comparison between sites in their average performance in the absence of treatment.
The point that matters here is that using the impact from the other site M (the length of the vertical line at M) to infer impact for my site N, leads to a result that is biased by an amount equal to the difference between the length of the vertical bars (Bias 1). But if I use the main approach that I am talking about, and compare performance under treatment at the remote site “M” (black bar at the top of Site M site) to the performance at my site without treatment (black bar at the bottom of Site N) the total bias is (Bias1 – Bias2), and the magnitude of this “net bias” is less than Bias1 by itself.
RE: Well, you have not figured-in the sampling error.
ER: Correct. We can do that, but for now let’s consider that we’re working with true values.
RE: OK, let’s say for the moment I accept what you’re saying. What does it do to the order and logic that internal validity precedes external validity?
ER: That is the question. What does it do? It seems that when generalizability is a concern, internal and external validity should be considered concurrently. Internal validity is the sole concern only when external validity is not at issue. You might say internal validity wins the race, but only when it’s the only runner.
RE: You’re going down a philosophical wormhole. That can be dangerous.
ER: Alright, then let’s stop here (for now).
RE and ER walk happily down the conference hall to the bar where RE has a double Jack, neat, and ER tries the house red.
BTW, here is the full argument and mathematical demonstration of the idea. Please share on social and tag us (our social handles are in the footer below). We’d love to know your thoughts. A.J.