Classrooms and Districts: Breaking Down Silos in Education Research and Evidence
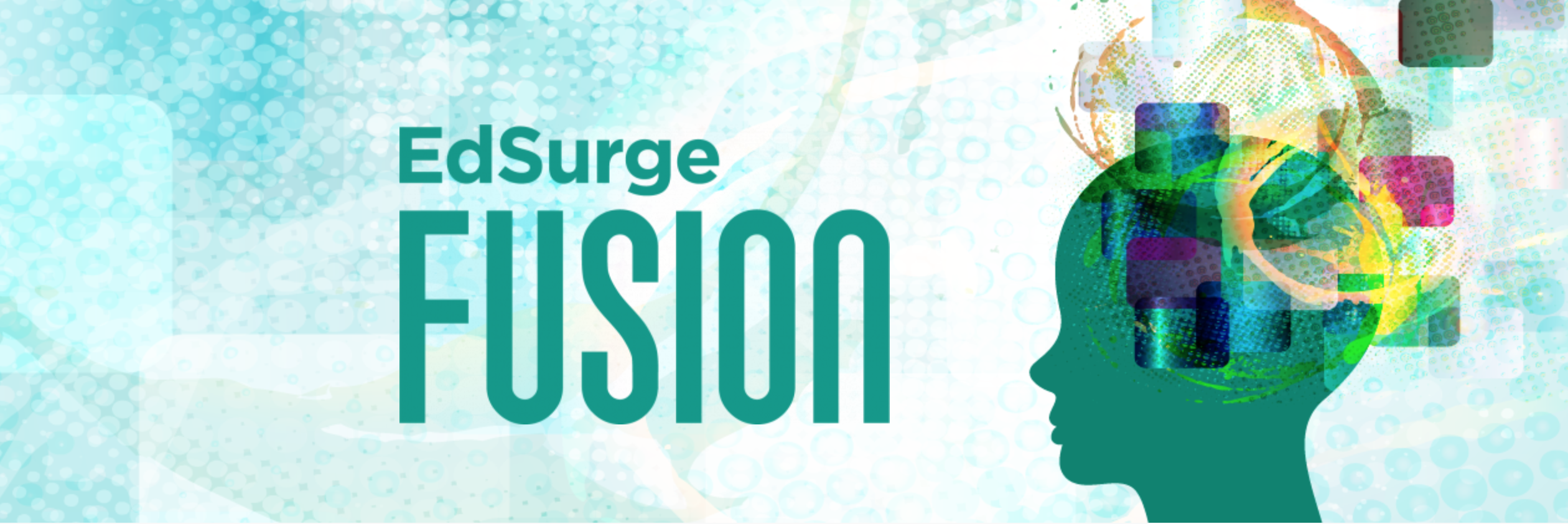
I just got back from Edsurge’s Fusion conference. The theme, aimed at classroom and school leaders, was personalizing classroom instruction. This is guided by learning science, which includes brain development and the impact of trauma, as well as empathetic caregiving, as Pamela Cantor beautifully explained in her keynote. It also leads to detailed characterizations of learner variability being explored at Digital Promise by Vic Vuchic’s team, which is providing teachers with mappings between classroom goals and tools and strategies that can address learners who vary in background, cognitive skills, and socio-emotional character.
One of the conference tracks that particularly interested me was the workshops and discussions under “Research & Evidence”. Here is where I experienced a disconnect between Empirical ’s research policy-oriented work interpreting ESSA and Fusion’s focus on improving the classroom.
- The Fusion conference is focused at the classroom level, where teachers along with their coaches and school leaders are making decisions about personalizing the instruction to students. They advocate basing decisions on research and evidence from the learning sciences.
- Our work, also using research and evidence, has been focused on the school district level where decisions are about procurement and implementation of educational materials including the technical infrastructure needed, for example, for edtech products.
While the classroom and district levels have different needs and resources and look to different areas of scientific expertise, they need not form conceptual silos. But the differences need to be understood.
Consider the different ways we look at piloting a new product.
- The Digital Promise edtech pilot framework attempts to move schools toward a more planful approach by getting them to identify and quantify the problem for which the product being piloted could be a solution. The success in the pilot classrooms is evaluated by the teachers, where detailed understandings by the teacher don’t call for statistical comparisons. Their framework points to tools such as the RCE Coach that can help with the statistics to support local decisions.
- Our work looks at pilots differently. Pilots are excellent for understanding implementability and classroom acceptance (and working with developers to improve the product), but even with rapid cycle tools, the quantitative outcomes are usually not available in time for local decisions. We are more interested in how data can be accumulated nationally from thousands of pilots so that teachers and administrators can get information on which products are likely to work in their classrooms given their local demographics and resources. This is where review sites like Edsurge product reviews or Noodle’s ProcureK12) could be enhanced with evidence about for whom, and under what conditions, the products work best. With over 5,000 edtech products, an initial filter to help choose what a school should pilot will be necessary.
A framework that puts these two approaches together is promulgated in the Every Student Succeeds Act (ESSA). ESSA defines four levels of evidence, based on the strength of the causal inference about whether the product works. More than just a system for rating the scientific rigor of a study, it is a guide to developing a research program with a basis in learning science. The base level says that the program must have a rationale. This brings us back to the Digital Promise edtech pilot framework needing teachers to define their problem. The ESSA level 1 rationale is what the pilot framework calls for. Schools must start thinking through what the problem is that needs to be solved and why a particular product is likely to be a solution. This base level sets up the communication between educators and developers about not just whether the product works in the classroom, but how to improve it.
The next level in ESSA, called “correlational,” is considered weak evidence, because it shows only that the product has “promise” and is worth studying with a stronger method. However, this level is far more useful as a way for developers to gather information about which parts of the program are driving student results, and which patterns of usage may be detrimental. Schools can see if there is an amount of usage that maximizes the value of the product (rather than depending solely on the developer’s rationale). This level 2 calls for piloting the program and examining quantitative results. To get correlational results, the pilot must have enough students and may require going beyond a single school. This is a reason that we usually look for a district’s involvement in a pilot.
The top two levels in the ESSA scheme involve comparisons of students and teachers who use the product to those who do not. These are the levels where it begins to make sense to combine a number of studies of the same product from different districts in a statistical process called meta-analysis so we can start to make generalizations. At these levels, it is very important to look beyond just the comparison of the program group and the control group and gather information on the characteristics of schools, teachers, and students who benefit most (and least) from the product. This is the evidence of most value to product review sites.
When it comes to characterizing schools, teachers, and students, the “classroom” and the “district” approach have different, but equally important, needs.
- The learner variability project has very fine-grained categories that teachers are able to establish for the students in their class.
- For generalizable evidence, we need characteristics that are routinely collected by the schools. To make data analysis for efficacy studies a common occurrence, we have to avoid expensive surveys and testing of students that are used only for the research. Furthermore, the research community must reach consensus on a limited number of variables that will be used in research. Fortunately, another aspect of ESSA is the broadening of routine data collection for accountability purposes, so that information on improvements in socio-emotional learning or school climate will be usable in studies.
Edsurge and Digital Promise are part of a west coast contingent of researchers, funders, policymakers, and edtech developers that has been discussing these issues. We look forward to continuing this conversation within the framework provided by ESSA. When we look at the ESSA levels as not just vertical but building out from concrete classroom experience to more abstract and general results from thousands of school districts, then learning science and efficacy research are combined. This strengthens our ability to serve all students, teachers, and school leaders.